The furious pace of modern research is creating a gnarly statistics problem
The truth is a needle in an ever-growing haystack. Scientists need better statistical education to find it
Imagine a hypothetical early scientist who wondered whether or not her tribe’s ritual rain dance had anything to do with whether or not it rained. Our scientist decides on the following simple and elegant experimental design: every day for a year, she wakes up and flips a coin. If it lands on heads, she performs a rain dance; if it lands on tails, she doesn't. At the end of the day, she records whether or not it rained.
Though a rain dance doesn't influence the weather, our scientist could, nevertheless, be incredibly unlucky. Perhaps, just by sheer chance, she finds that over the course of the entire year, it happened to rain 99 percent of the times she performed her rain dance. She might reasonably come to the false conclusion that her rain dance causes it to rain.
This is all to say: science is fallible. Even at its best, science can make mistakes. A good scientist will design her experiment so that the chances of arriving at a false conclusion are low, but she can never design a perfect experiment; she always has to live with some small, lingering chance that what looked like compelling data was actually just plain happenstance.
Most reasonable people will begrudgingly accept that any given scientific finding has a small chance of being false. It's like finding out that the FDA allows 1mg of mouse feces in a pound of black pepper; mouse poop is unsavory, but at least it only makes up 1 part in 450,000. However, there has been a growing concern for a bit over a decade that the state of science is far worse than this. Perhaps most famously, Stanford professor John Ioannidis proclaimed in 2005: “It can be proven that most claimed research findings are false.” Though the disease is severe, the root cause is unassuming. Some statisticians, like Ioannidis, suspect that the primary culprit is simply a widespread confusion about statistics.
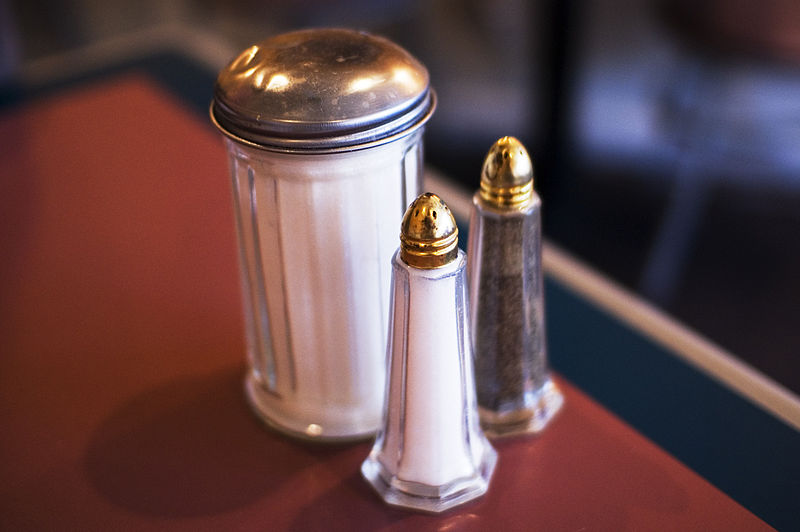
Pick your poison
Every day we rely on the careful work of scientists in ways both big and small. From the ability to send an emoji halfway across the world to the countless miracles of modern medicine, the mechanics of our everyday lives constantly involve the fruits of scientific labor. But those innovations are just a small slice of scientific inquiry happening daily, and proof that some science works for us shouldn't blind us to the field's shortcomings.
A burglary in Penn Station
Look at it like this: Suppose you are in Penn Station, in New York City, at 5 pm: it's rush hour, and 100,001 people are whizzing by. A masked burglar commits a mugging and, in an effort to catch the thief, the police immediately lock all of the doors. Though nobody got a great look at the burglar, we can be sure that he is in there somewhere.
The only hope of catching him is if the criminal left a partial fingerprint on his victim’s purse. Each and every commuter is fingerprinted, and they sit and wait while the evidence is shuttled off to a lab for processing.
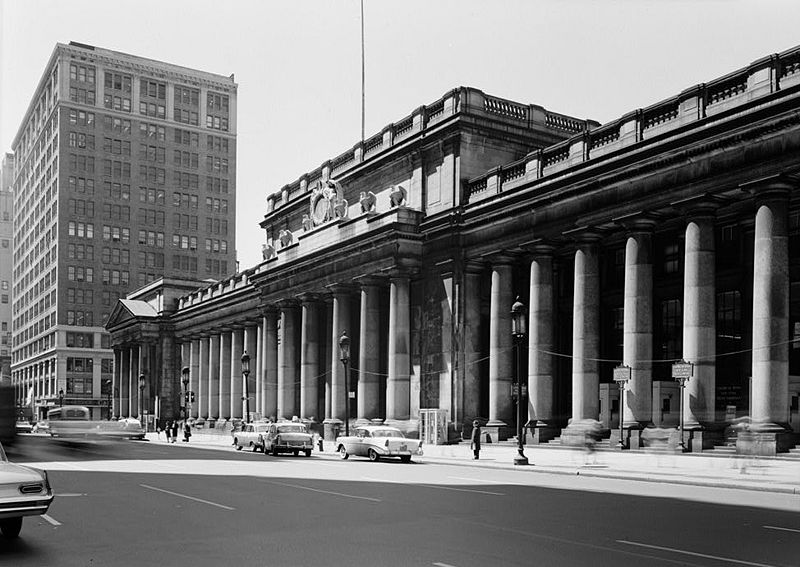
Penn Station, 1962. It used to be nice
There is a 1 percent chance that an innocent person’s fingerprint will accidentally match the fingerprint found at the crime. Since there were 100,000 innocent bystanders, we should expect around 1,000 false matches to be found. Mixed in with these innocent suspects will be the one true criminal, now just a drop in an ocean of 1,001 suspects. Even though there was only a 1 percent chance that the fingerprinting technology mistakenly matched an innocent person, the probability that any one of the 1,001 primary suspects is innocent is a whopping 99.9%.
That is all to say, there are far more incorrect hypotheses about how the world works than there are correct ones. The truth is a needle in a haystack; this is precisely what makes science so hard. Like an innocent bystander in Penn Station, any incorrect hypothesis might be mistaken for the truth. Because there are so many hypotheses that scientists test, many scientific findings — even most — could be mistakes. In statistical parlance, this is known as the problem of “multiple hypothesis testing.”
Solving the rain dance question
Statisticians have been aware of this danger for quite awhile. In 1957, D.V. Lindley wrote a paper that outlined almost the exact same argument. But it's particularly relevant now, because scientific practice has changed drastically since the early 2000s.
Today, testing a scientific hypothesis has become immensely easier due to various technological advances. For instance, improvements in DNA sequencing technology has made it possible for modern biologists to regularly test millions of hypotheses in one shot. Whereas scientists of old had to be somewhat discerning about the hypotheses they chose to test, modern scientists can easily cast a broad net and just test them all. This made common the conditions of our Penn Station thought experiment: many innocent bystanders intermingle with extremely rare culprits.
I should mention that statisticians are not all in lock-step with this idea. Estimates of the proportion of published scientific discoveries that are false is a hotly debated issue. While Ioannidis thinks it possible that the vast majority of published findings are false, Professor Jeffery Leek of University of Washington puts the figure much lower, estimating in an interesting 2014 paper that about 14 percent of scientific findings are likely to be false. However, as Ioannidis and others would quickly point out, there are some serious reasons to suspect that this estimate is too small.
For every scientific finding we hear about, lurking in its wake is a trail of negative results that we never hear about. Rather than stating the results of every experiment they run, scientists only tell us the results of the interesting ones. This bashfulness has severe statistical consequences. To come back to our Penn Station analogy, it obfuscates the number of commuters locked in the station. This opacity matters. Whether there are 100,001 commuters or 101 commuters changes our proportion of false discoveries from 99.99 percent to just 50 percent.
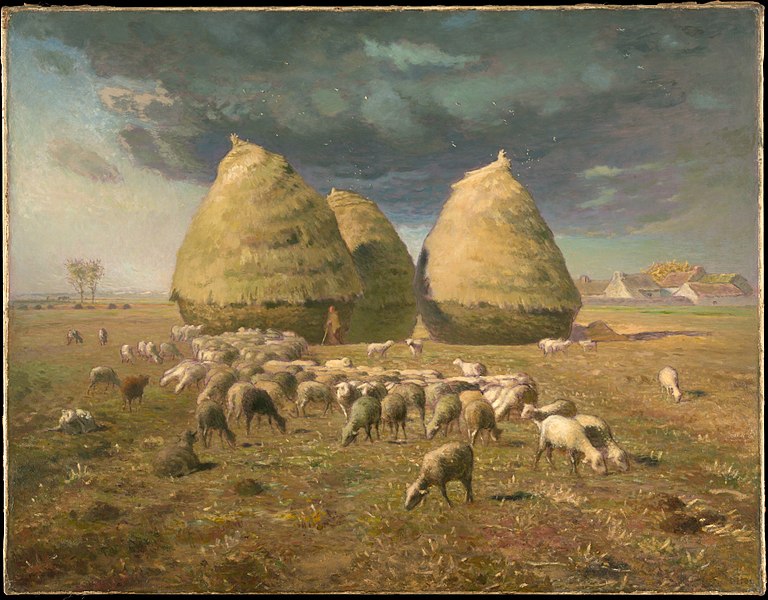
Spot the truth
There are many compelling reasons to push for publishing "uninteresting" or "negative" results. Efficiency is one obvious reason: scientists need not waste time testing hypotheses that have been repeatedly shown to be false. Pruning false results is another reason. Even if our early scientist discovered that dancing causes it to rain, another scientist, repeating her experiment, will be unlikely to find the same. Without the publication of this second, negative result, the belief that dancing causes it to rain could long remain a "scientific finding" for far too long. But perhaps most importantly, without negative results, evaluating whether a purported scientific discovery is true is simply impossible.
However imperfect and error-prone science is, it remains our best tool at getting to the truth. The thing is, scientists are wrong so often not because they are clumsy, lying, or stupid. Scientists are wrong so often because the questions they ask are difficult ones — scientists seek truth, and truth is rare and elusive.
Because the root cause of so many false scientific discoveries is widespread statistical confusion, a solution is feasible: statistical education. The science community is, even if slowly, recognizing the necessity of modifying its statistical practices to suit modern scientific research. Perhaps one day soon we will be able to say triumphantly: “Most scientific discoveries are true.” Today, however, to assume that scientists are always right, or even usually right, is naive.
As someone who went to MIT and took three statistics courses (one through the math department, one through the computer science department, and one through the brain and science department) and STILL doesn't feel like I've internalized a solid understanding of stats, how would you suggest improving statistics pedagogy?