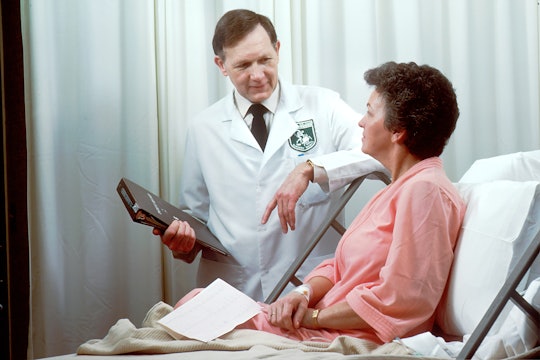
NCI via Unsplash
Artificial intelligence can help diagnose skin cancer, but only on white skin
A new image-based AI tool can suggest clinical next steps for melanoma, but for darker skinned patients, equal outcomes are lacking
When people see a dermatologist, they are normally concerned about some kind of area of disease on the skin — especially an area that looks suspiciously cancerous. The doctor will examine the area and, in some cases, will take a biopsy to determine what type of disease it is (if any). Armed with this newfound information, dermatologists are left to determine how best to proceed. However, this process is not as efficient as it might seem.
In the dermatology field, there is a persistent disconnect between the diagnosis of what a skin disease is and how it is managed. This can sometimes occur when diagnoses are incorrect, which prompts dermatologists to suggest an incorrect course of action for managing the disease. However, even if the diagnosis is correct, what, if any, clinical management steps are taken next are left up to the doctor. For example, if someone is diagnosed with skin cancer, doctors might order a clinical follow-up to confirm the diagnosis, they might plan an appointment to get the growth excised, or they might decide that no immediate action is needed. Because this step is highly subjective, any two dermatologists might take different action when presented with the same patient, and they might even make mistakes that lead to worse patient outcomes.
A recent study published in Scientific Reports proposes a new artificial intelligence (AI) tool that could act as a second opinion for dermatologists when considering the best course of action for following up on potentially cancerous skin spots. However, whether this will solve any of the systemic problems in dermatology clinical management remains to be seen.
The tool, created by researchers Kumar Abhishek, Jeremy Kawahara, and Ghassan Hamarneh at Simon Fraser University, predicts appropriate clinical management steps based on an image of a diseased area of skin. While other AI models have been taught to diagnose skin spots, this would be the first to prioritize clinical management instead. In other words, this tool "looks" at an image of skin then recommends either a clinical follow-up, immediate excision, or no action.
To create this tool, the researchers designed a software program using publicly available datasets with images of diseased skin. As the authors fed the model more skin images, it learned to recognize clinically-relevant features of the skin spots such as asymmetric borders, color, and size. While these are often indicators of malignant rather than benign skin disease, they are not specific to any singular cancer type. Once trained, their AI model was able to sort images based on relevant clinical features and predict what clinical management step should be taken.
The researchers found that their tool had a high level of accuracy that matched the consensus of dermatologists. Researchers allowed the tool to assess 100 skin photos, then compared the model's outputs to the recommendations of 157 dermatologists using the same photoset. Statistically, the model had better agreement with the aggregated recommendations of all dermatologists than the levels of agreement between any two dermatologists with one another.
However, the successful proof-of-concept tool comes with a caveat: while it was tested on multiple image datasets, almost none of the images included photos of brown or Black people's skin. This is a huge problem, as BIPOC have lower overall survival rates for skin cancer. In fact, the five-year survival rate for non-white patients with melanoma is 20 percent lower than that of white patients.
This is largely because many skin diseases, particularly skin cancers, present differently on non-white patients and physicians are not adequately trained to identify these diseases in a diverse patient population. This makes misdiagnoses common for BIPOC and leads to them getting wrong or delayed treatment. Because of this, those with darker skin are more than twice as likely to present with late-stage or metastatic melanoma than white people.
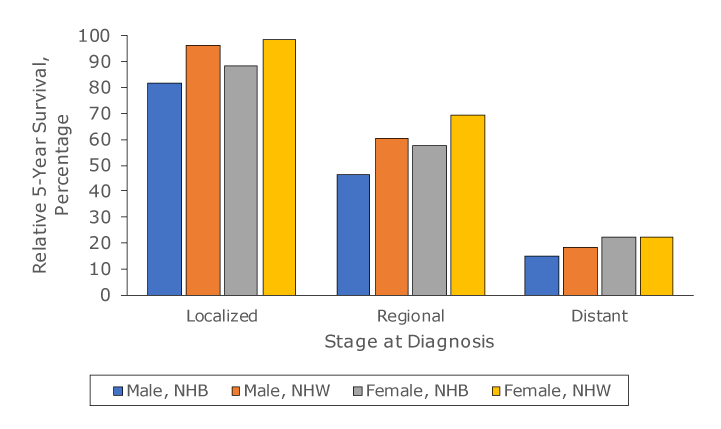
A chart showing lower survival rates for non-Hispanic Black (NHB) and non-Hispanic white (NHW) patients regardless of early (local), middle (regional), or late-stage (distant) progression
Via CDC
Technology that could effectively act as a “second opinion” and prioritize clinical management over diagnosis would be invaluable to BIPOC people and those in other underserved communities who might not have access to highly trained and experienced dermatologists. However, without training new potential software programs with non-white samples, this type of technology is unlikely to be effective in the populations that need it most.
AI is powerful, and has the capacity to make our lives, both in healthcare and beyond, better. However, the results of this study exemplify a known problem in AI tech that’s more than skin deep — AI is inherently racially biased. The datasets that are fed to software programs reflect the systemic racism inherent in our human world. When using publicly available datasets that do not reflect real-world populations, AI tends to perpetuate human problems rather than solve them.
Moving forward, we must prioritize using racially diverse datasets to train AI programs. Further, the dermatology field must focus on increasing diversity both among their workforce and in the datasets they use to train doctors. Dermatology is the second least ethnically diverse medical field, with Hispanic and Black dermatologists comprising only 4.2 percent and 3 percent of the workforce, respectively. Lastly, researchers and companies who develop AI tools should aim to minimize discrimination in their software. By prioritizing equity in AI, or at the very least ensuring that active efforts are being taken to reduce discrimination in AI and in healthcare, we can begin to create tools that can operate at their highest potential and do the most good.
Excellent write up, Gloria! It highlights how important it is to look closely at the data that is used to train AI models. On hindsight, it is not surprising that this tool did not work on non-white skin, as the training data set was 100 skin images of predominantly white patients. Considering the lack of publicly available data sets for non–white patients, I wonder if it is worth introducing ‘skin tone’ as a variable factor, and training the model to recognize clinically-relevant features, as they have done now, for a variety of digitally manipulated skin tones. This is a simplistic view of the problem, but may help to create a skin tone neutral version of the tool.