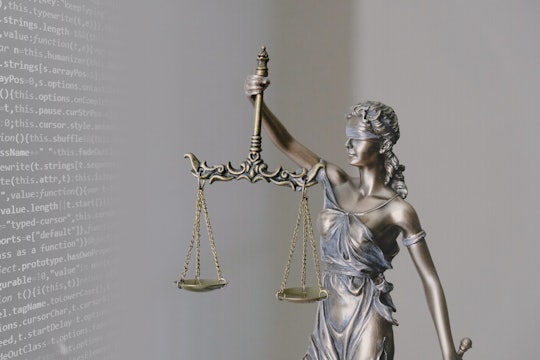
B.G. Borowiec, adapted from Roozbeh Eslami and Tingey Injury Law Firm on Unsplash
Can the criminal justice system's artificial intelligence ever be truly fair?
Computer programs used in 46 states incorrectly label Black defendants as “high-risk” at twice the rate as white defendants
In 2016, ProPublica reported that an artificial intelligence tool used in courtrooms across the United States to predict future crimes, the Correctional Offender Management Profiling for Alternative Sanctions (COMPAS), was biased against Black defendants.
COMPAS takes into account factors such as previous arrests, age, and employment. Its output — risk scores for recidivism — are one of the factors judges use to determine whether defendants will face jail or prison time. Using COMPAS, Black defendants were incorrectly labeled as “high-risk” to commit a future crime twice as often as their white counterparts. Northpointe, the parent company of COMPAS, refuted ProPublica’s claims, stating that the algorithm was working as intended.
Northpointe argued that Black people have a higher baseline risk of committing future crimes after an arrest — and this results in higher risk scores for Black people as a group. Northpointe, now rebranded as Equivant, hasn't publicly changed the way it computes risk assessments.
Computer science researchers have tried to fix racist algorithms by imposing a set of criteria they’ve dubbed “fairness.” Research on fairness — the mathematical criteria that researchers place on algorithms to ensure that outcomes aren't based on race — has grown, with Amazon alone putting 20 million dollars into it last year. But a coherent picture of fairness in the field remains frustratingly elusive.
The answer may lie in simply changing how we define "fairness."
Experts in algorithmic fairness worry the current approaches to defining fairness will never result in actual fair outcomes for Black people. They warn that a purely mathematical solution focused on "accuracy" is a myopic approach to deciding what is fair. Accuracy, in the context of fairness, is a measure of how correct an algorithm's predictions are. But an algorithm used to predict a future crime will only reinforce the logic of the carceral system, where some people are always more likely to be deemed high-risk because of how law enforcement treats race. Solving this problem means considering the outcomes of the use of an algorithm, the impact of oppression, and tackling the inherent unfairness in society at its root.
“[The] discourse has largely been motivated by a concern that there’s no convergence on a single notion of what would make an algorithmic system fair,” says Lily Hu, a PhD candidate in applied mathematics at Harvard University who studies algorithmic fairness. Hu argues that the reason for this is because the use of algorithms in social spaces, particularly in the prison system, is an inherently political problem, not a technological one. “There are societal problems where technological solutions are great," Hu says, "like indoor plumbing."
"But many of the problems we’re trying to solve," Hu continues, "aren’t problems of that kind.”
The use of artificial intelligence algorithms in predictive policing and risk assessments is only growing despite continuous reporting that these tools often perpetuate harsher sentencing of Black defendants and harsher policing of Black communities. Risk assessments, like COMPAS, are commonly used in pretrial detention. They’re often marketed as methods to aid in criminal justice reform across jurisdictions in the United States. They are used in 46 states in the US.
Because of their many problems, many criminal justice experts caution against the use of risk assessments in criminal justice reform. Yet to proponents, the appeal of artificial intelligence algorithms in place of a human decision-maker in assessments is clear: they allow law-makers, justices, and police officers to shift responsibility onto a seemingly more objective actor. Proponents argue that algorithms are a way of overcoming the social biases of human decision-makers, citing their increased "accuracy" (compared to humans) as an asset. They continue to be adopted in jurisdictions across the US.
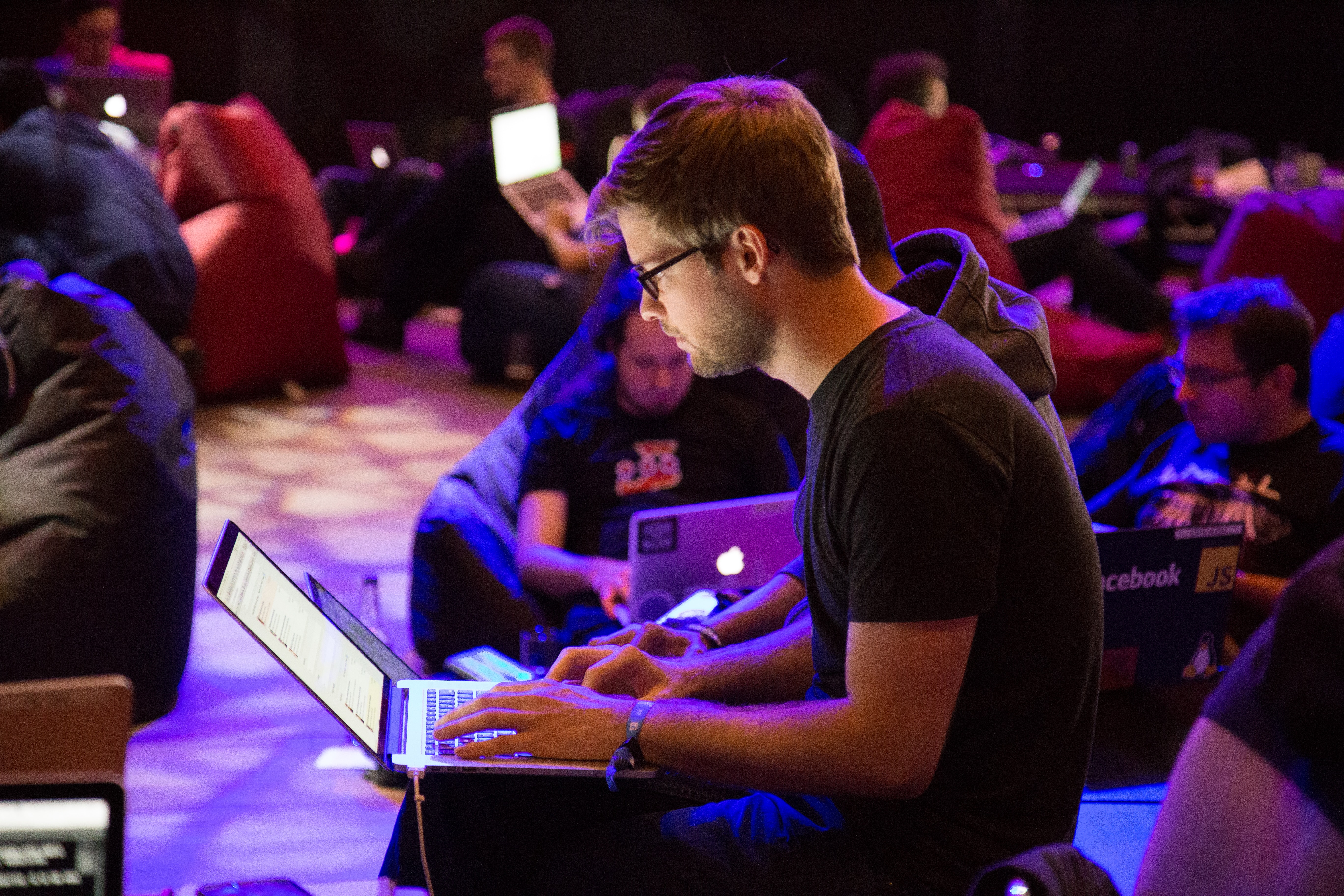
"Garbage in, garbage out." Algorithms reflect the biases of their creators, who are typically white men.
Alex Kotliarskyi on Unsplash
To call risk assessment algorithms objective is to ignore the social systems and structures in which they are created. It is clear now that artificial intelligence algorithms can carry the biases of their mostly-white programmers. They base predictions on data, which can be incomplete or carry socially-ingrained stereotypes and prejudices. Both sources of bias can lead to bad predictions that hurt people of color. Recent, high profile examples include Facebook face-recognition algorithms mislabeling photos of Black people as gorillas, or mistakenly labeling Asian people as blinking in photos.
In the previous cases (and within the context of social media), the solution might be to build fairer, more "accurate" algorithms, trained on more representative data. In the case of criminal justice, experts argue that even perfect algorithms — those with 100 percent accuracy — will still perpetuate systemic bias and end up hurting people of color. Inaccurate predictions lead to bias, but accurate ones reinforce existing bias.
“The underlying problem here is that we have racialized hierarchies of what crime means and who is at 'risk' of committing those crimes (not to mention who is at risk of being caught for those crimes), combined with a policy that punishes those at high risk with the loss of liberty,” writes Ben Green, a Professor at the University of Michigan who studies algorithmic fairness, in an email to Massive. “Even if risk assessments could attain some type of perfect prediction, that wouldn’t solve the problem. A perfect risk assessment could have the perverse effect of making this policy appear more legitimate and making those who are detained as a result appear to be more truly deserving of that punishment."
For true "fairness" — true equality for marginalized people — the prison system must abandon the use of algorithms in risk assessments altogether.
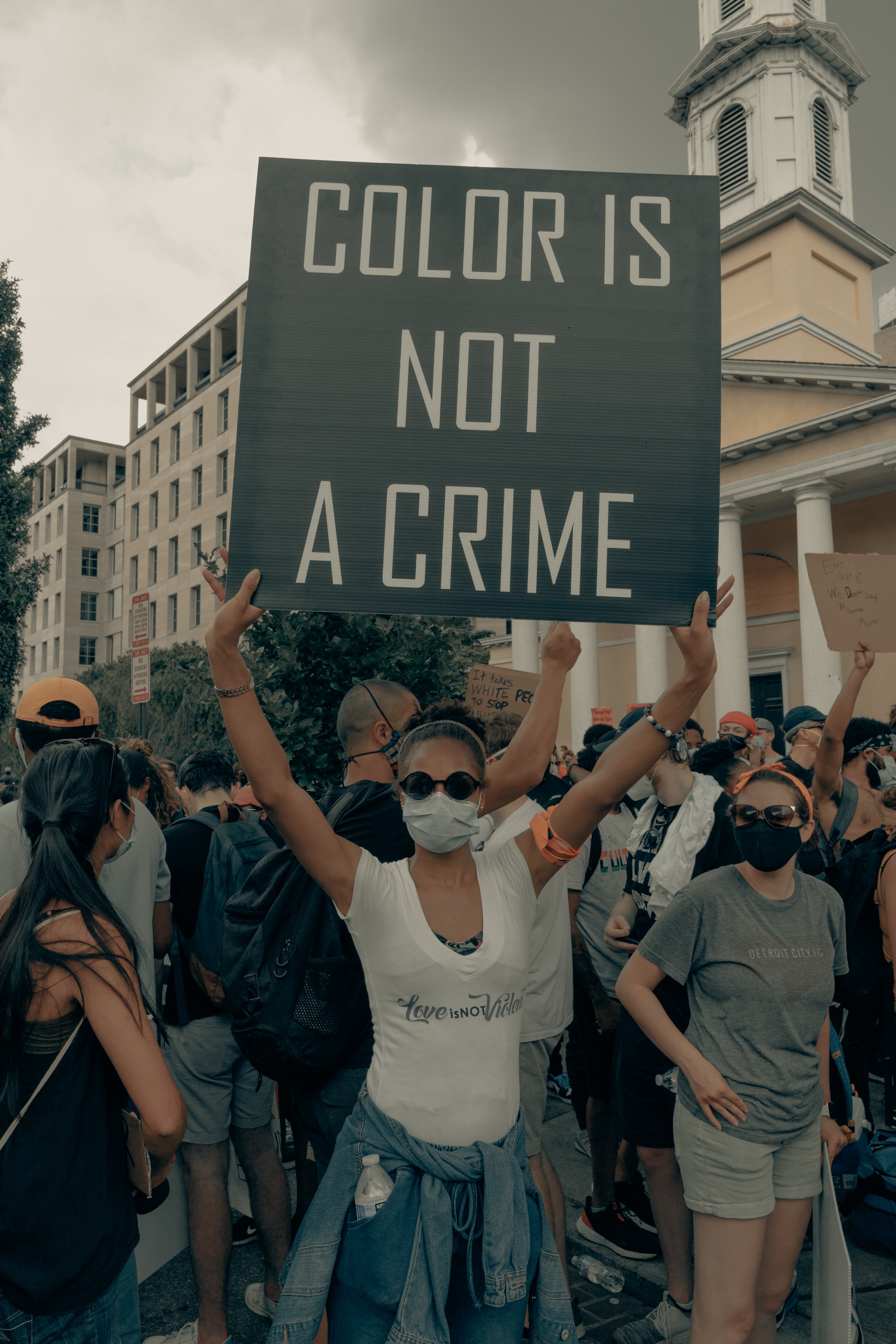
A person holds up sign ("Color is not a crime") at the Black Lives Matter protest in Washington DC Unsplash
Clay Banks via Unsplash
Much of the tension (including the ProPublica-Northpointe debate) around fairness circles around the inherent incompatibility around two major mathematical definitions of fairness. One, called calibration, supposes that what an algorithm predicts to be the actual underlying risk that someone will reoffend (50% chance of reoffending means 50% chance of rearrest, regardless of the offender's race). Another is that the number of errors be equal for both groups. Both definitions can't be satisfied at once. This (statistically proven) incompatibility has been dubbed the “impossibility of fairness” and has led some researchers to conclude that implementing algorithms requires inherent, unavoidable trade-offs. Others have argued that calibration is sufficient and algorithms like COMPAS, which meet the calibration criteria, are fair.
Hu argues that the impossibility of fairness is just illustrating the political and ethical nature of the problem. “The impossibility of fairness just shows in mathematical terms that we can’t have it all,” she says.
According to Green, the problem is that two common notions of fairness don’t properly tackle the unfairness imposed by social inequality. Algorithms meant to ensure that offenders remain imprisoned fail to account for the systemic reasons why more Black people may end up in jail and reoffend. Such narrow definitions aren’t promoting just outcomes — they're perpetuating broken definitions of what justice is.
“The fundamental problem here is not actually that there are different definitions of fairness that could be used. The problem is that our focus is incredibly narrow, considering only a single decision point. If we think about the two major fairness notions, neither really get to the heart of the matter of social hierarchy and institutionalized inequality. We need to take a broader view of the matter — and by doing so we can also realize that the impossibility of fairness doesn’t provide as intractable of a dilemma as is typically assumed,” writes Green.
According to Green, it isn’t clear that we should assume that we want algorithms to work to increase the number of offenders in jail or prison. Incarceration is in itself harmful, and has long-term negative impacts. We can think about mathematical fairness all we want, but instead, we should just abolish jails and prisons.
Interesting article! It’s also important to note that these algorithms are trained using a stand-in measure of the actual outcome - so “likelihood of being convicted of a crime” is often treated as an accurate measure of “likelihood of committing a crime” by the people developing the algorithm. I think this is mentioned in the article but it’s one of the key reasons these kind of algorithms will always be racist - they are being trained with the goal of accurately predicting the decisions of an inherently racist system.